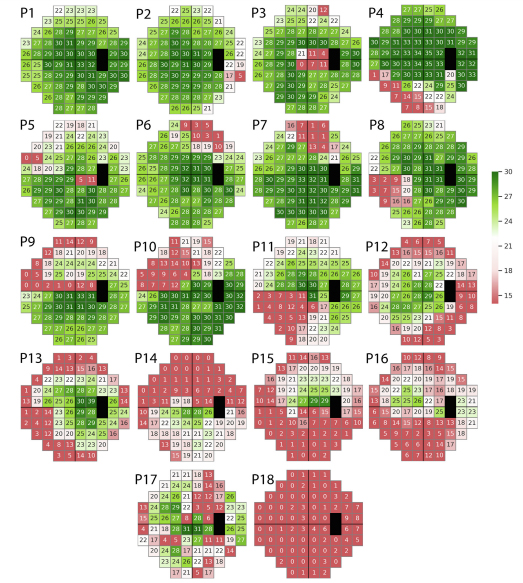
Glaucoma progression
Purpose: To identify patterns of visual field (VF) loss based on unsupervised machine learning and to identify patterns that are associated with rapid progression.
Conclusions: An automated machine learning system can identify patterns of VF loss and could provide objective and reproducible nomenclature for characterizing early signs of visual defects and rapid progression in patients with glaucoma.
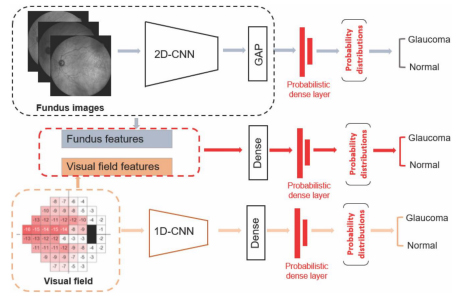
Detecting glaucoma from multi-modal data
Purpose: To assess the accuracy of probabilistic deep learning models to discriminate normal eyes and eyes with glaucoma from fundus photographs and visual fields.
Conclusions: Probabilistic deep learning models can detect glaucoma from multi-modal data with high accuracy. Our findings suggest that models based on combined visual field and fundus photograph modalities detect glaucoma with higher accuracy. While probabilistic and deterministic CNN models provided similar performance, probabilistic models generate a certainty level of the outcome thus providing another level of confidence in decision-making.

Detecting retinal neural and stromal cell classes and ganglion cell subtypes based on transcriptome data
Purpose: To develop and assess the accuracy of deep learning models that identify different retinal cell types, as well as different retinal ganglion cell (RGC) subtypes, based on patterns of single-cell RNA sequencing (scRNA-seq) in multiple datasets.
Conclusions: We developed a deep-learning-based domain adaptation model to detect retinal cell types and RGC subtypes from transcriptome profiles. The model was highly accurate in detecting correct RGC subtypes and was resistant to the batch effect. Further validation of our model holds promise for future studies to identify transcriptome profiles of RGCs and other cells involved in glaucoma to shed light on molecular aspects of glaucoma pathogenesis.